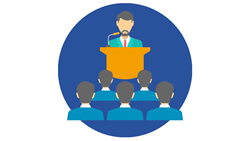
CRAN - ENSEM - 7 mars - 14h - amphi Gallé
Gautman Biswas, Professeur invité (Vanderbilt University) : Combining Data-Driven and Model-Based Methods to Improve Diagnosis of Complex Systems and Control
Gautman Biswas, Professeur invité (Vanderbilt University) : Combining Data-Driven and Model-Based Methods to Improve Diagnosis of Complex Systems and Control
Abstract:
Traditional methods for model-based diagnosis assume an equation-based or a graphical model can be constructed to represent system behavior. This model forms the basis for designing fault detection and isolation schemes. However, as systems have become more complex, and with the advent of embedded and cyber physical systems (CPS), it is becoming harder to construct models of systems that are sufficiently complete and accurate to support correct diagnoses. On the other hand, recent advances in sensor technology in conjunction with the computing revolution has enabled significant scaling of data collection, data storage, and data processing schemes, leading researchers and practitioners to now turn their attention to data-driven methods for fault detection and isolation. In this talk, I will outline different ways in which data (especially large amounts of operational data) can be used by itself or combined with models to achieve more accurate and more complete diagnosis systems.
After presenting a framework for combining model- and data-driven methods for diagnosis, I will present a number of case studies from work we have done in enhancing model-based diagnosers with data-driven methods. Then I will discuss our more recent work in using unsupervised learning methods for anomaly detection, i.e., finding previously undetected faults from large volumes of operational data collected from complex systems. Last, I will present reinforcement learning methods we have been developing toward data-driven control and fault-adaptive control of complex systems.
Bio:
Gautam Biswas holds an Endowed Chair – he is a Cornelius Vanderbilt Professor of Computer Science and Computer Engineering, in the EECS Department and a Senior Research Scientist at the Institute for Software Integrated Systems (ISIS) at Vanderbilt University. He has an undergraduate degree in Electrical Engineering from the Indian Institute of Technology (IIT) in Mumbai, India, and M.S. and Ph.D. degrees in Computer Science from Michigan State University in E. Lansing, MI. Currently, Prof. Biswas is the lead on the VISOR (Vanderbilt Initiative for Smart cities Operations and Research) TIPS center at Vanderbilt University.
Prof. Biswas conducts research in Intelligent Systems with primary interests in hybrid modeling, simulation, and analysis of complex embedded systems, and their applications to diagnosis, prognosis, and fault-adaptive control. More recently, he is working on data mining for diagnosis, and developing methods that combine model-based and data-driven approaches for diagnostic and prognostic reasoning. This work, in conjunction with Honeywell Technical Center and NASA Ames, received the NASA 2011 Aeronautics Research Mission Directorate Technology and Innovation Group Award for Vehicle Level Reasoning System and Data Mining methods to improve aircraft diagnostic and prognostic systems. In other research projects, he is involved in developing simulation-based environments for learning and instruction. His research has been supported by funding from NASA, NSF, DARPA, and the US Department of Education. He has over 600 refereed publications, and is a Fellow of the IEEE, a Prognostics and Health Management (PHM) Society Fellow, and member of the ACM, AAAI, AIED, and the Sigma Xi Research Societies.